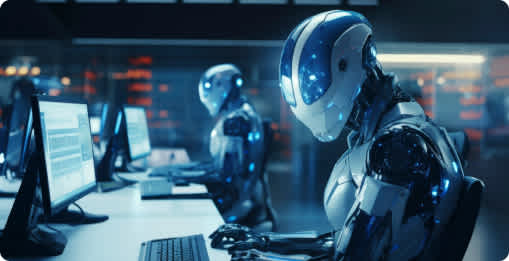
The Insanity of Relying on Vector Embeddings: Why RAG Fails
Wrong Tool for the Job
RAG fails in production because vector embeddings are the wrong choice for determining percentage of sameness. This is easily demonstrated. Consider the following three words:
King
Queen
Ruler
King and ruler can refer to the same person (and are thus considered synonyms). But king and queen are distinctly different people. From the perspective of percentage of sameness, king/ruler should have a high score and king/queen should be literally zero.In other words, if the query is asking something about a “king” then chunks discussing a “queen” would be irrelevant; but chunks discussing a “ruler” might be relevant. Yet, vector embeddings consider “queen” to be more relevant to a search on “king” than “ruler.” Here are the vector similarity scores for queen and ruler when compared to king using OpenAI’s ADA-002 embeddings:
King
Queen: 92%
Ruler: 83%